Fundamentals in Neural Networks
Build up your intuition of the fundamental building blocks of Neural Networks.
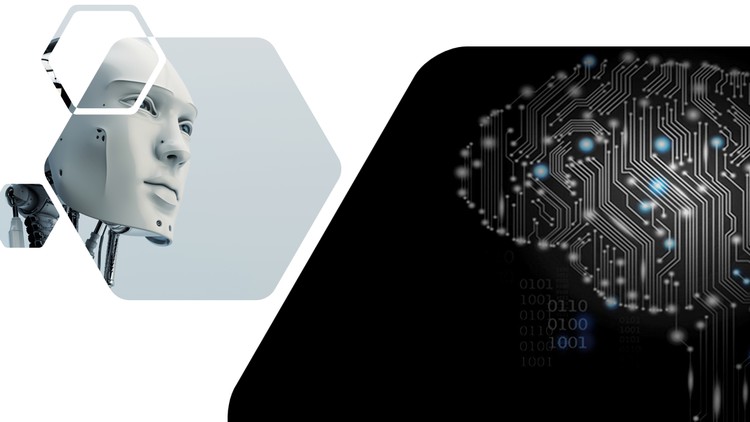
What you will learn
Understand the intuition behind Artificial Neural Networks
Understand the intuition behind Convolutional Neural Networks
Understand the intuition behind Recurrent Neural Networks
Apply Artificial Neural Networks in practice
Apply Convolutional Neural Networks in practice
Apply Recurrent Neural Networks in practice
Why take this course?
Deep learning (also known as deep structured learning) is part of a broader family of machine learning methods based on artificial neural networks with representation learning. Learning can be supervised, semi-supervised or unsupervised. Deep-learning architectures such as deep neural networks, deep belief networks, deep reinforcement learning, recurrent neural networks and convolutional neural networks have been applied to fields including computer vision, speech recognition, natural language processing, machine translation, bioinformatics, drug design, medical image analysis, material inspection and board game programs, where they have produced results comparable to and in some cases surpassing human expert performance.
This course covers the following three sections: (1) Neural Networks, (2) Convolutional Neural Networks, and (3) Recurrent Neural Networks. You will be receiving around 4 hours of materials on detailed discussion, mathematical description, and code walkthroughs of the three common families of neural networks. The descriptions of each section is summarized below.
Section 1 - Neural Network
1.1 Linear Regression
1.2 Logistic Regression
1.3 Purpose of Neural Network
1.4 Forward Propagation
1.5 Backward Propagation
1.6 Activation Function (Relu, Sigmoid, Softmax)
1.7 Cross-entropy Loss Function
1.8 Gradient Descent
Section 2 - Convolutional Neural Network
2.1 Image Data
2.2 Tensor and Matrix
2.3 Convolutional Operation
2.4 Padding
2.5 Stride
2.6 Convolution in 2D and 3D
2.7 VGG16
2.8 Residual Network
Section 3 - Recurrent Neural Network
3.1 Welcome
3.2 Why use RNN
3.3 Language Processing
3.4 Forward Propagation in RNN
3.5 Backpropagation through Time
3.6 Gated Recurrent Unit (GRU)
3.7 Long Short Term Memory (LSTM)
3.8 Bidirectional RNN (bi-RNN)
Section 4 - Technical Walkthrough: Artificial Neural Network
This section walks through each and every building block of deploying an Artificial Neural Network using tensorflow.
Section 5 - Technical Walkthrough: Convolutional Neural Network
This section walks through each and every building block of deploying a Convolutional Neural Network using tensorflow.
Section 6 - Technical Walkthrough: Recurrent Neural Network
This section walks through each and every building block of deploying an Recurrent Neural Network using tensorflow.
Section 7 - Advanced Topics: Autoencoders
This section walks through each and every building block of deploying an Autoencoder using tensorflow. Further, we explore the inference problems using the latent layers of the autoencoder.
Section 8 - Advanced Topics: Image Segmentation
This section walks through each and every building block of deploying an Image-to-image model using tensorflow.
Screenshots
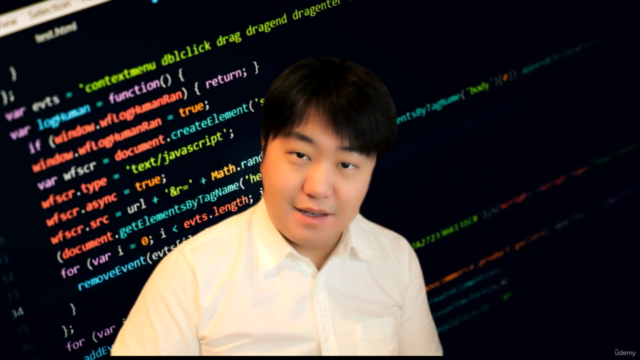
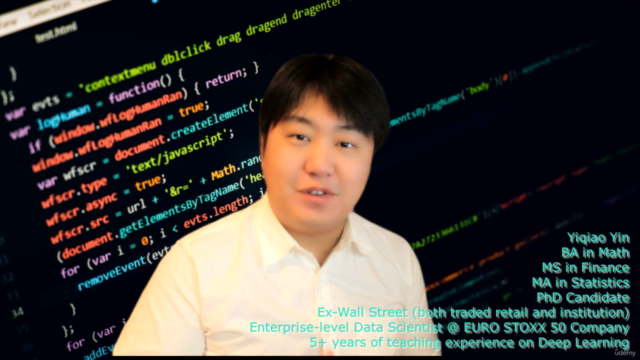
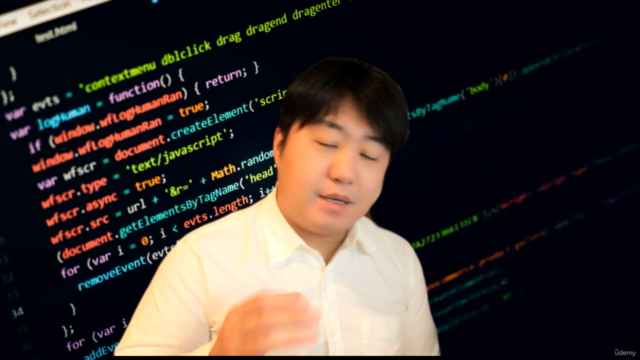
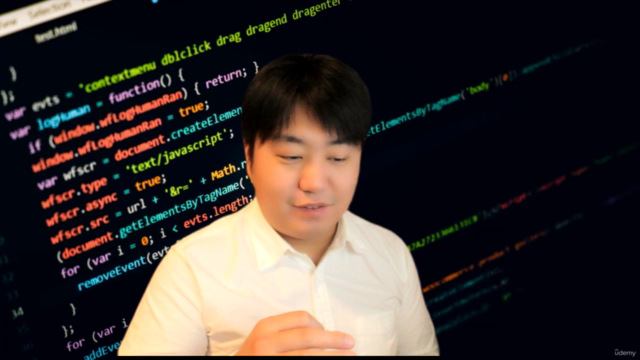
Reviews
Charts
Price
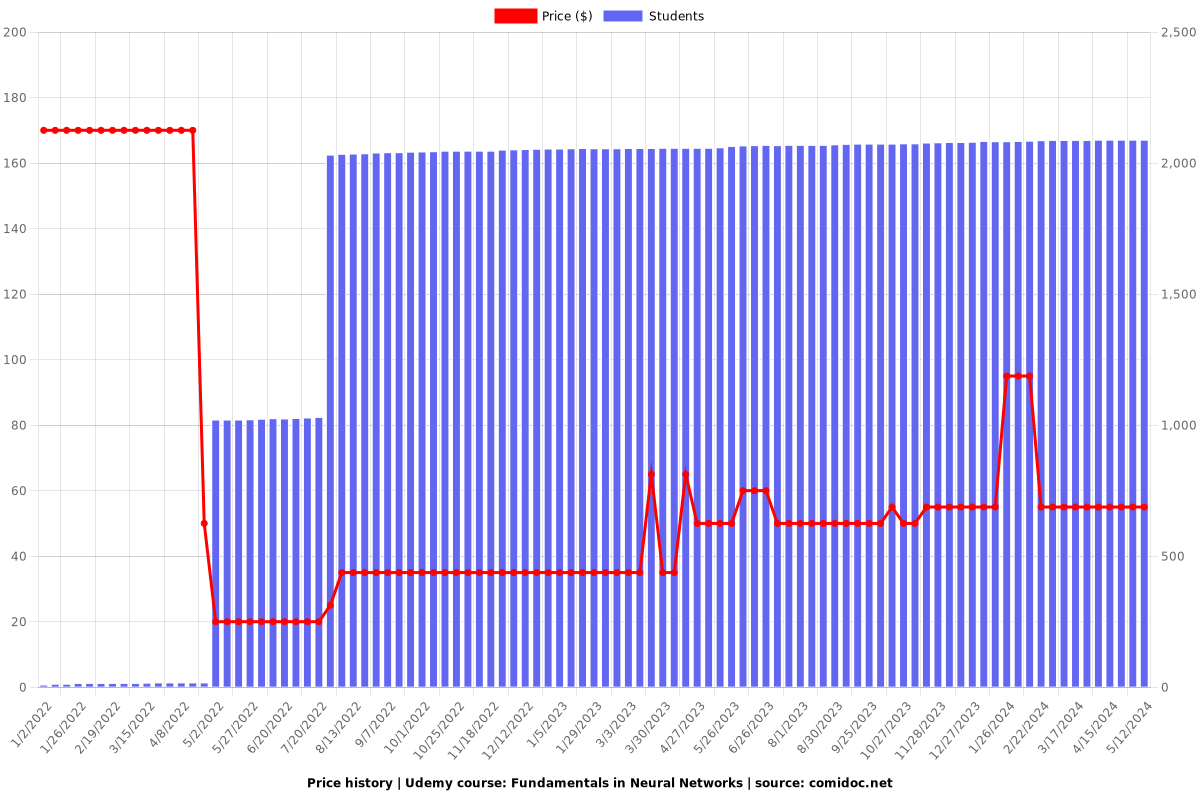
Rating
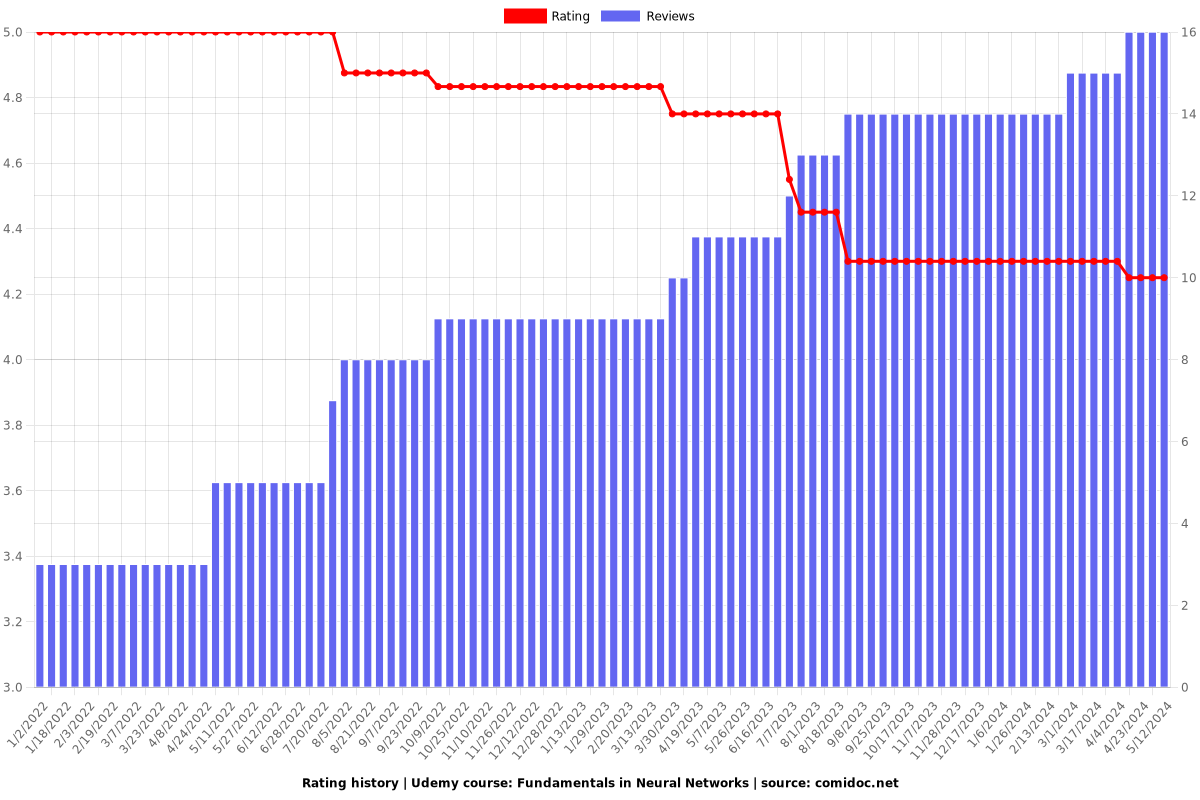
Enrollment distribution
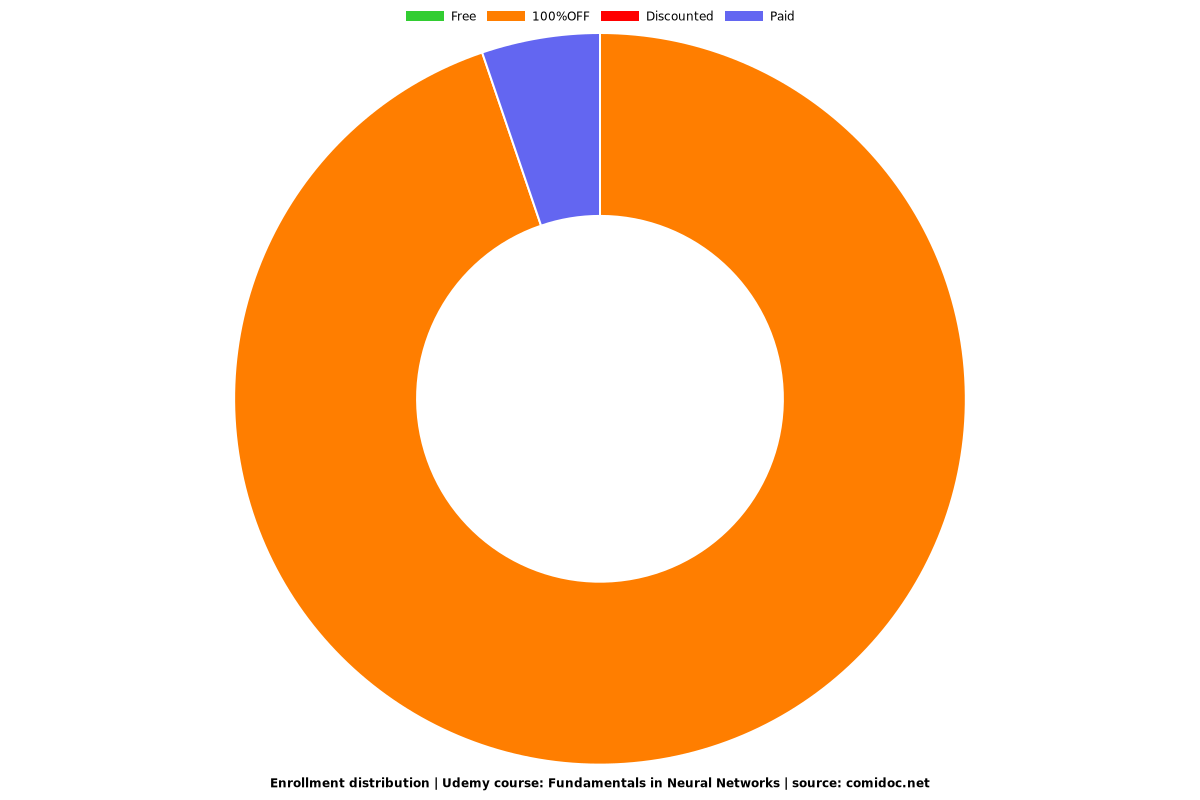