Visualization and Imputation of Missing Data
Learn to create numerous unique visualizations to better understand patterns of missing data in your data sample.
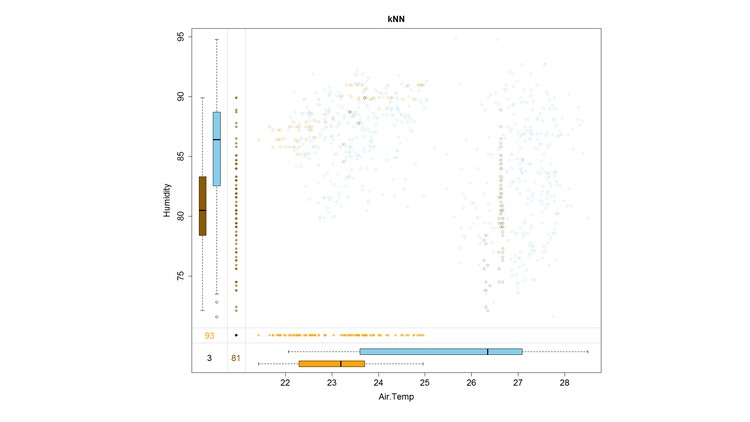
What you will learn
Use visualizations created by R software to identify patterns of 'missingness' in data sets and to impute reasonable values to replace the missing data.
Recognize and identify the different patterns of missing data and the relative severity of their likely consequences.
Learn to use the VIM and VIMGUI R packages to create unique, novel and vibrant images which promote the understanding of patterns of both missing and imputed data in a set of data.
Learn the different historical approaches to impute reasonable values for missing data and their relative advantages and disadvantages.
Learn the characteristics of: (1) Hot-Deck; (2) K-Nearest Neighbor; (3) Regression-Based; and (4) Iterative, Model-Based, Stepwise Regression (IRMI) imputation techniques to "fill in" missing data and when and how to implement them with provided software.
Why take this course?
There are many problems associated with analyzing data sets that contain missing data. However, there are various techniques to 'fill in,' or impute, missing data values with reasonable estimates based on the characteristics of the data itself and on the patterns of 'missingness.' Generally, techniques appropriate for imputing missing values in multivariate normal data and not as useful when applied to non-multivariate-normal data. This Visualization and Imputation of Missing Data course focuses on understanding patterns of 'missingness' in a data sample, especially non-multivariate-normal data sets, and teaches one to use various appropriate imputation techniques to "fill in" the missing data. Using the VIM and VIMGUI packages in R, the course also teaches how to create dozens of different and unique visualizations to better understand existing patterns of both the missing and imputed data in your samples.
The course teaches both the concepts and provides software to apply the latest non-multivariate-normal-friendly data imputation techniques, including: (1) Hot-Deck imputation: the sequential and random hot-deck algorithm; (2) the distance-based, k-nearest neighbor imputation approach; (3) individual, regression-based imputation; and (4) the iterative, model-based, stepwise regression imputation technique with both standard and robust methods (the IRMI algorithm). Furthermore, the course trains one to recognize the patterns of missingness using many vibrant and varied visualizations of the missing data patterns created by the professional VIMGUI software included in the course materials and made available to all course participants.
This course is useful to anyone who regularly analyzes large or small data sets that may contain missing data. This includes graduate students and faculty engaged in empirical research and working professionals who are engaged in quantitative research and/or data analysis. The visualizations that are taught are especially useful to understand the types of data missingness that may be present in your data and consequently, how best to deal with this missing data using imputation. The course includes the means to apply the appropriate imputation techniques, especially for non-multivariate-normal sets of data which tend to be most problematic to impute.
The course author provides free-of-charge with the course materials his own unique VIMGUI toolbar developed in the RGtk2 visualization programming language in R. However, please note that both the R-provided VIMGUI package (developed in RGtk2), as well as the course author's provided VIMGUI toolbar application (also developed in RGtk2) may have some problems starting up properly on a Mac computer. So if you only have a Mac available to you, you may have some initial difficulties getting the applications to run properly.
Screenshots
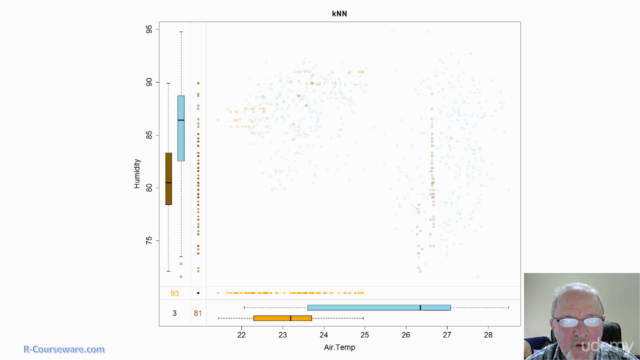
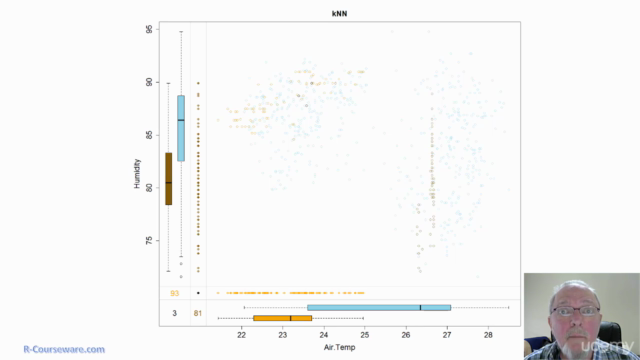
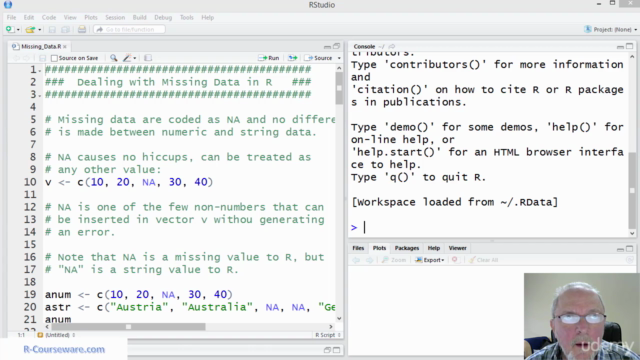
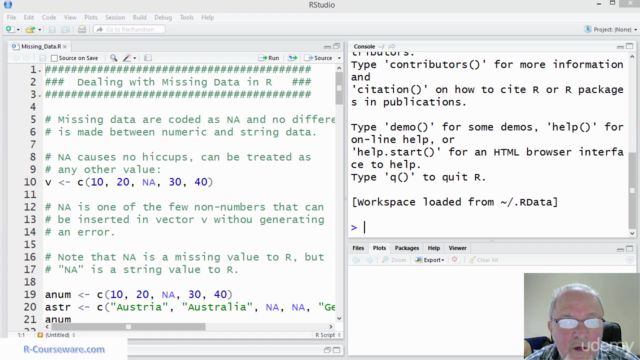
Reviews
Charts
Price
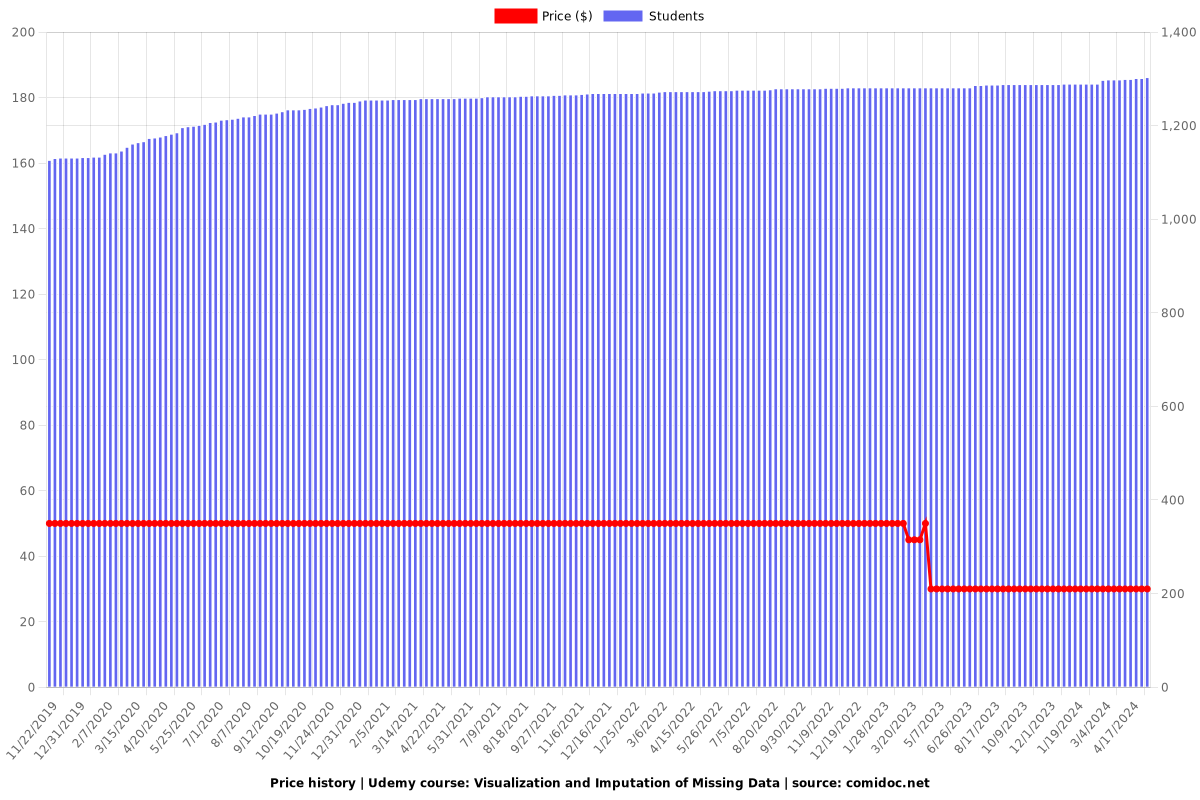
Rating
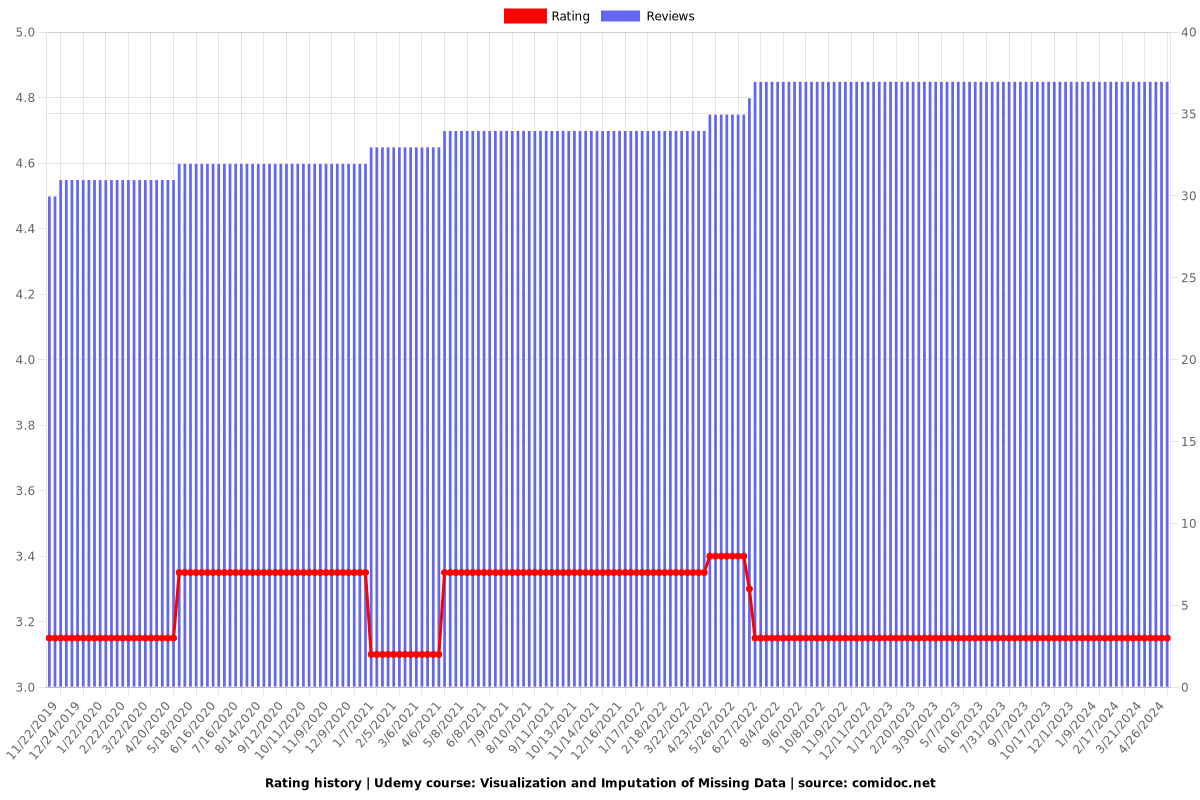
Enrollment distribution
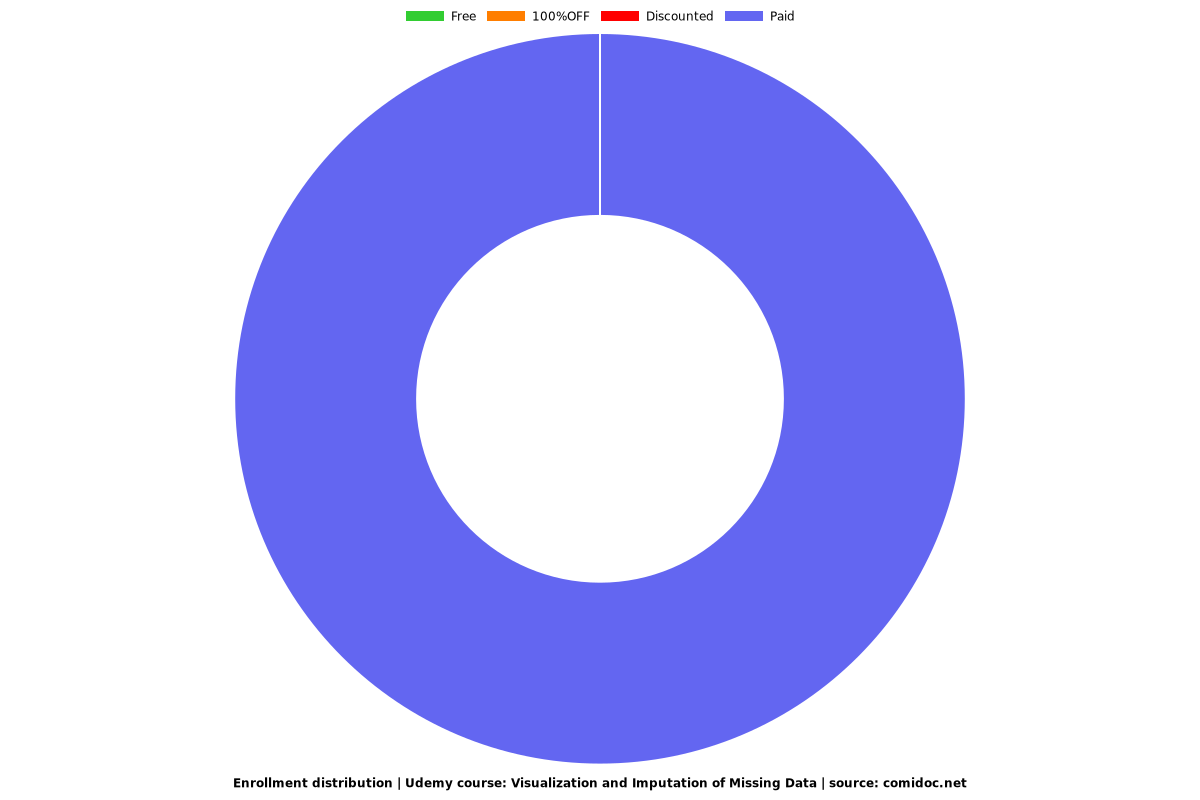