Supervised Machine Learning Principles and Practices-Python
Algorithms and Practical Examples in Python
4.18 (19 reviews)
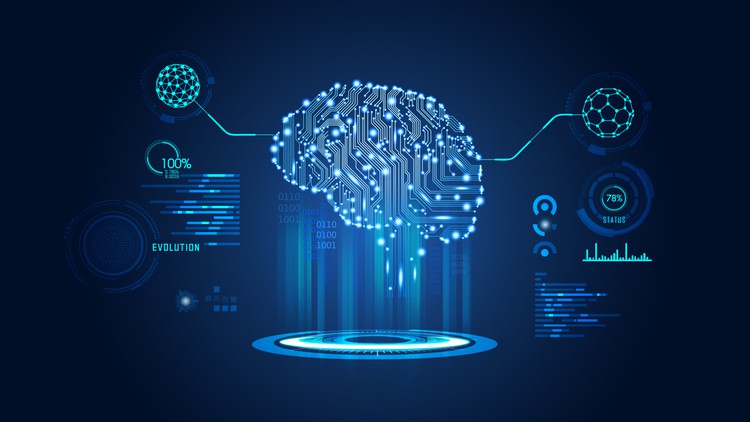
396
students
5.5 hours
content
Mar 2023
last update
$19.99
regular price
What you will learn
Understand the mathematics behine Machine Learning
Supervised Machine Learning Models such as Decision Tree, Support Vector Machine, k-Nearest Neighbor, Linear Regression etc.
Python Code for Supervised learning models
Creating a ML model and solving for a given set of data.
Related Topics
4950066
udemy ID
10/28/2022
course created date
4/5/2023
course indexed date
Bot
course submited by