Supervised Learning for AI with Python and Tensorflow 2
Uncover the Concepts and Techniques to Build and Train your own Artificial Intelligence Models
4.70 (37 reviews)
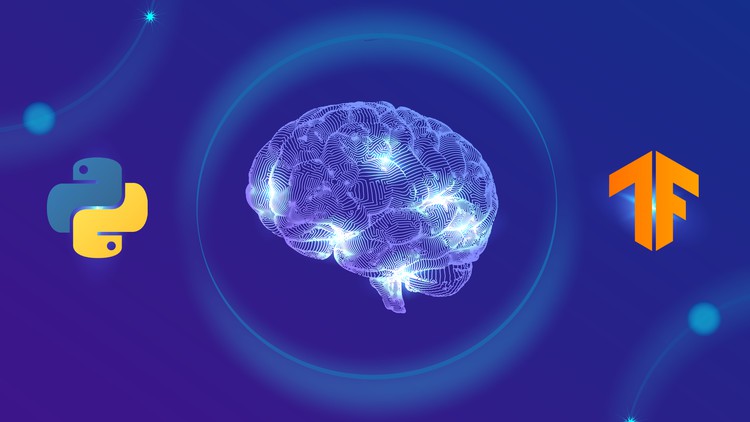
3,572
students
21 hours
content
Nov 2021
last update
$64.99
regular price
What you will learn
The basics of supervised learning: What are parameters, What is a bias node, Why do we use a learning rate
Techniques for dealing with data: How to Split Datasets, One-hot Encoding, Handling Missing Values
Vectors, matrices and creating faster code using Vectorization
Mathematical concepts such as Optimization, Derivatives and Gradient Descent
Gain a deep understanding behind the fundamentals of Feedforward, Convolutional and Recurrent Neural Networks
Build Feedforward, Convolutional and Recurrent Neural Networks using only the fundamentals
How to use Tensorflow 2.0 and Keras to build models, create TFRecords and save and load models
Practical project: Style Transfer - Use AI to draw an image in the style of your favorite artist
Practical project: Object Detection - Use AI to Detect the bounding box locations of objects inside of images
Practical project: Transfer Learning - Learn to leverage large pretrained AI models to work on new datasets
Practical project: One-Shot Learning - Learn to build AI models to perform tasks such as Face recognition
Practical project: Text Generation - Build an AI model to generate text similar to Romeo and Juliet
Practical project: Sentiment Classification - Build an AI model to determine whether text is overall negative or positive
Practical project: Attention Model - Build an attention model to build an interpretable AI model
Screenshots
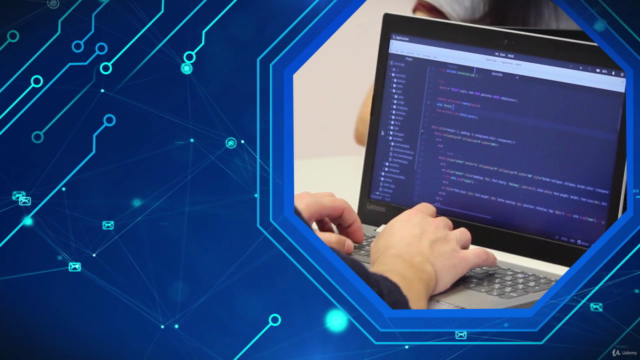
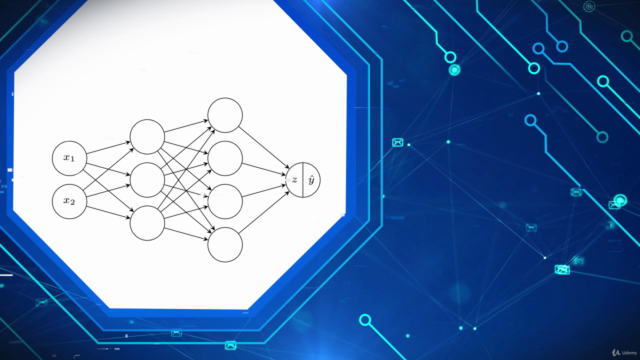
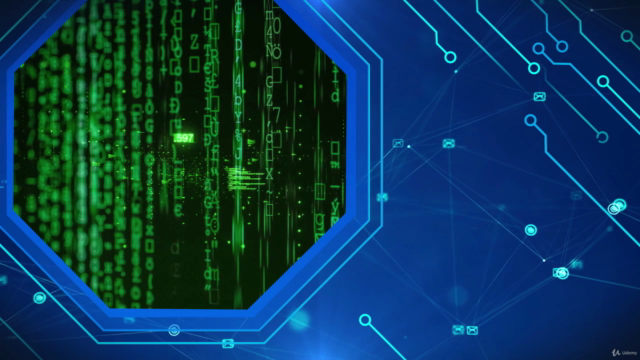
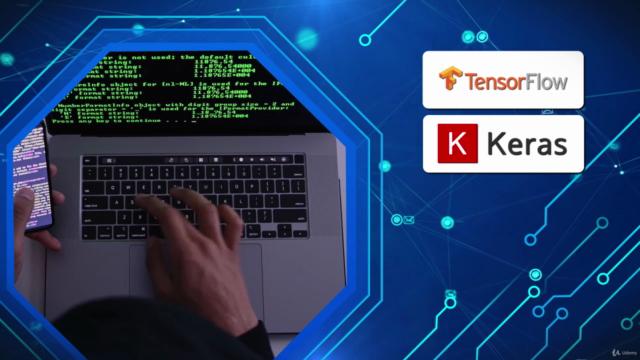
Related Topics
3926436
udemy ID
3/20/2021
course created date
6/11/2021
course indexed date
Bot
course submited by