Neural Networks in Python: Deep Learning for Beginners
Learn Artificial Neural Networks (ANN) in Python. Build predictive deep learning models using Keras & Tensorflow| Python
4.49 (1447 reviews)
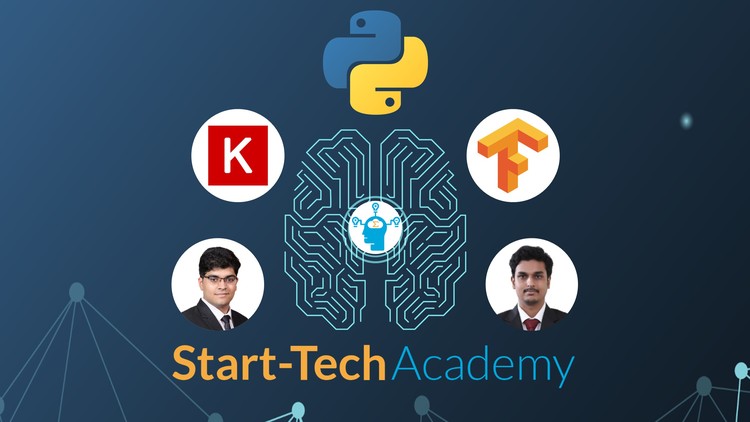
130,163
students
9.5 hours
content
Mar 2025
last update
$84.99
regular price
What you will learn
Get a solid understanding of Artificial Neural Networks (ANN) and Deep Learning
Understand the business scenarios where Artificial Neural Networks (ANN) is applicable
Building a Artificial Neural Networks (ANN) in Python
Use Artificial Neural Networks (ANN) to make predictions
Learn usage of Keras and Tensorflow libraries
Use Pandas DataFrames to manipulate data and make statistical computations.
Screenshots
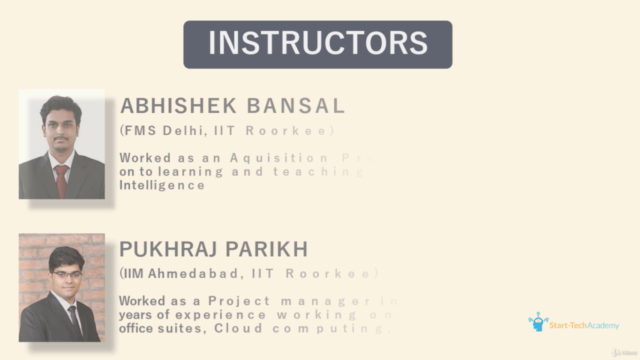
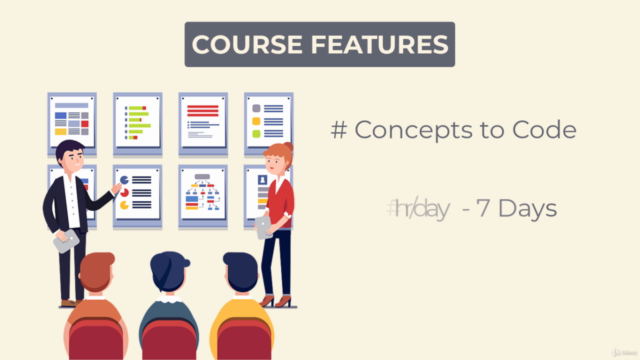
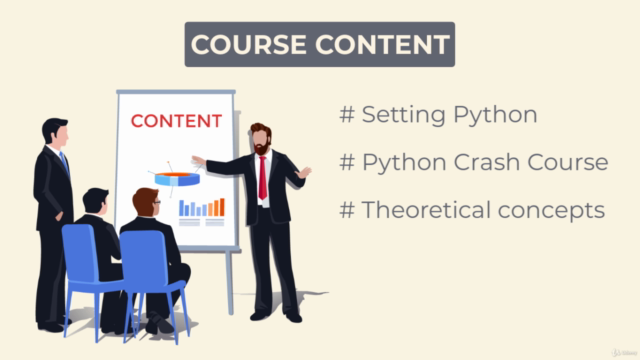
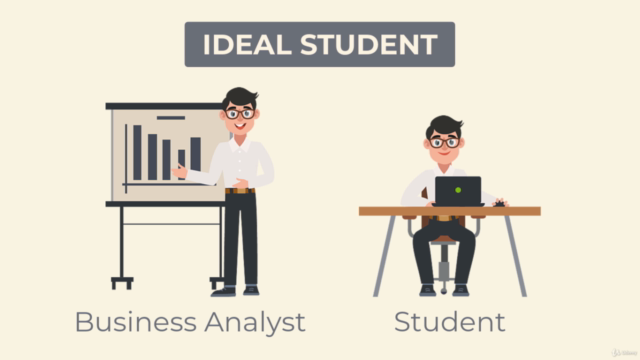
Related Topics
2652356
udemy ID
11/12/2019
course created date
11/16/2019
course indexed date
Bot
course submited by