Google Cloud Professional Data Engineer: Get Certified 2022
Build scalable, reliable data pipelines, databases, and machine learning applications.
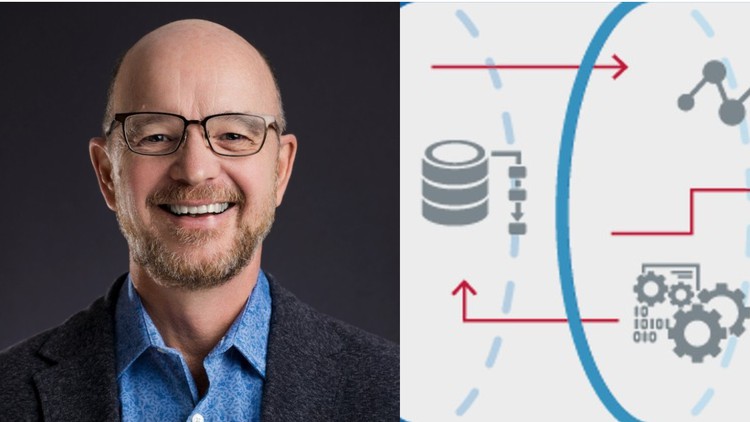
What you will learn
How to pass the Google Cloud Professional Data Engineer Exam
Build scalable, reliable data pipelines
Choose appropriate storage systems, including relational, NoSQL and analytical databases
Apply multiple types of machine learning techniques to different use cases
Deploy machine learning models in production
Monitor data pipelines and machine learning models
Design scalable, resilient distributed data intensive applications
Migrate data warehouse from on-premises to Google Cloud
Evaluate and improve the quality of machine learning models
Grasp fundamental concepts in machine learning, such as backpropagation, feature engineering, overfitting and underfitting.
Why take this course?
The need for data engineers is constantly growing and certified data engineers are some of the top paid certified professionals. Data engineers have a wide range of skills including the ability to design systems to ingest large volumes of data, store data cost-effectively, and efficiently process and analyze data with tools ranging from reporting and visualization to machine learning. Earning a Google Cloud Professional Data Engineer certification demonstrates you have the knowledge and skills to build, tune, and monitor high performance data engineering systems.
This course is designed and developed by the author of the official Google Cloud Professional Data Engineer exam guide and a data architect with over 20 years of experience in databases, data architecture, and machine learning. This course combines lectures with quizzes and hands-on practical sessions to ensure you understand how to ingest data, create a data processing pipelines in Cloud Dataflow, deploy relational databases, design highly performant Bigtable, BigQuery, and Cloud Spanner databases, query Firestore databases, and create a Spark and Hadoop cluster using Cloud Dataproc.
The final portion of the course is dedicated to the most challenging part of the exam: machine learning. If you are not familiar with concepts like backpropagation, stochastic gradient descent, overfitting, underfitting, and feature engineering then you are not ready to take the exam. Fortunately, this course is designed for you. In this course we start from the beginning with machine learning, introducing basic concepts, like the difference between supervised and unsupervised learning. We’ll build on the basics to understand how to design, train, and evaluate machine learning models. In the process, we’ll explain essential concepts you will need to understand to pass the Professional Data Engineer exam. We'll also review Google Cloud machine learning services and infrastructure, such as BigQuery ML and tensor processing units.
The course includes a 50 question practice exam that will test your knowledge of data engineering concepts and help you identify areas you may need to study more.
By the end of this course, you will be ready to use Google Cloud Data Engineering services to design, deploy and monitor data pipelines, deploy advanced database systems, build data analysis platforms, and support production machine learning environments.
ARE YOU READY TO PASS THE EXAM? Join me and I'll show you how!
Reviews
Charts
Price
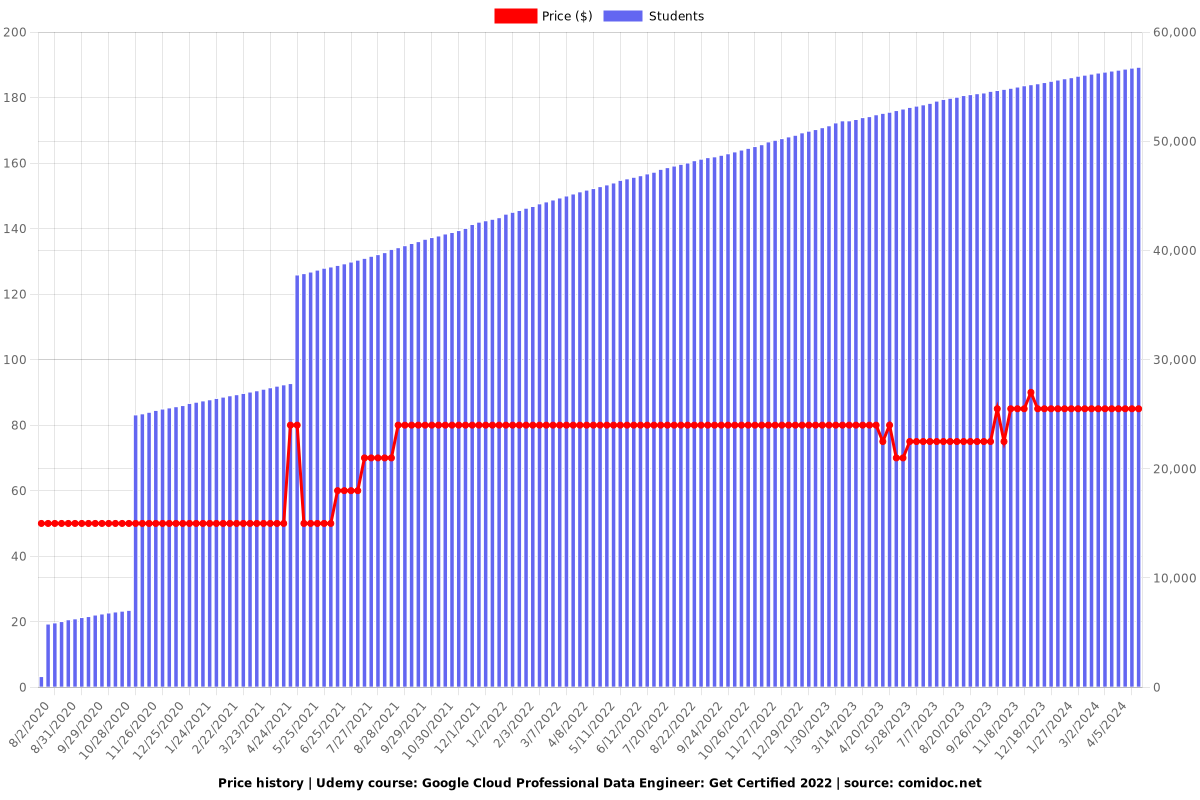
Rating
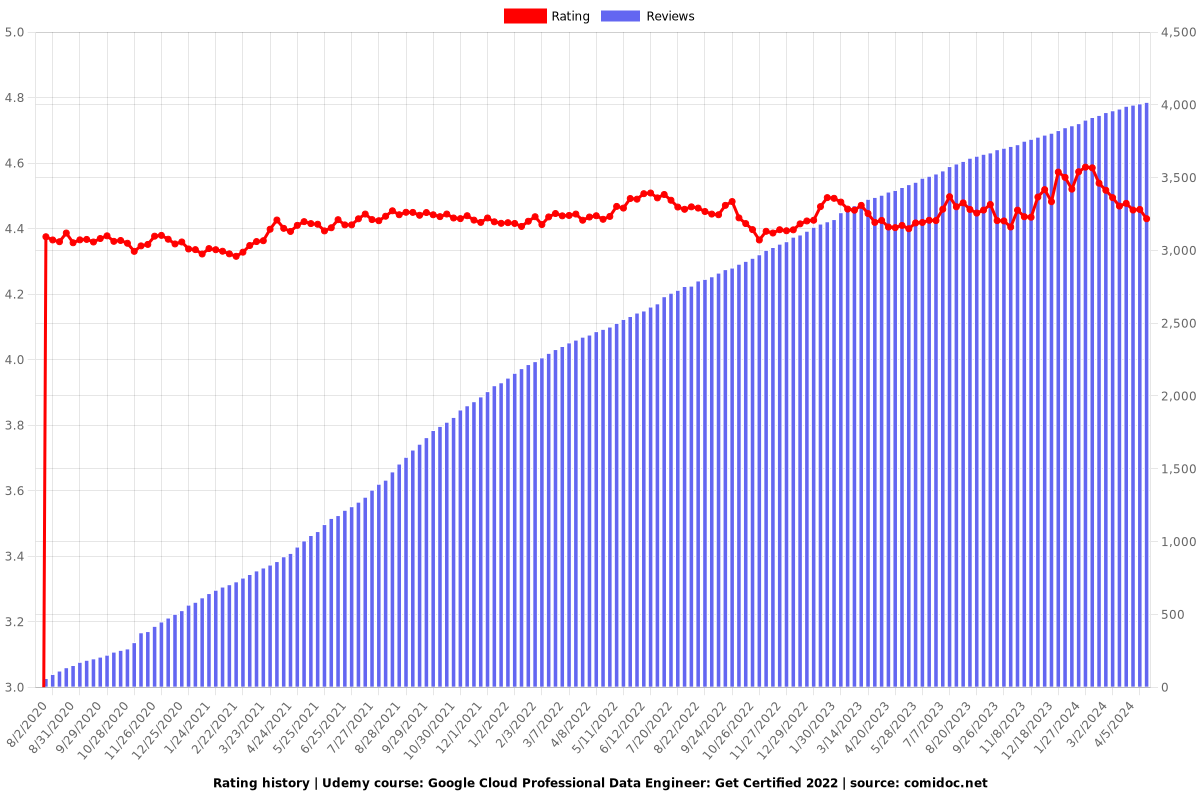
Enrollment distribution
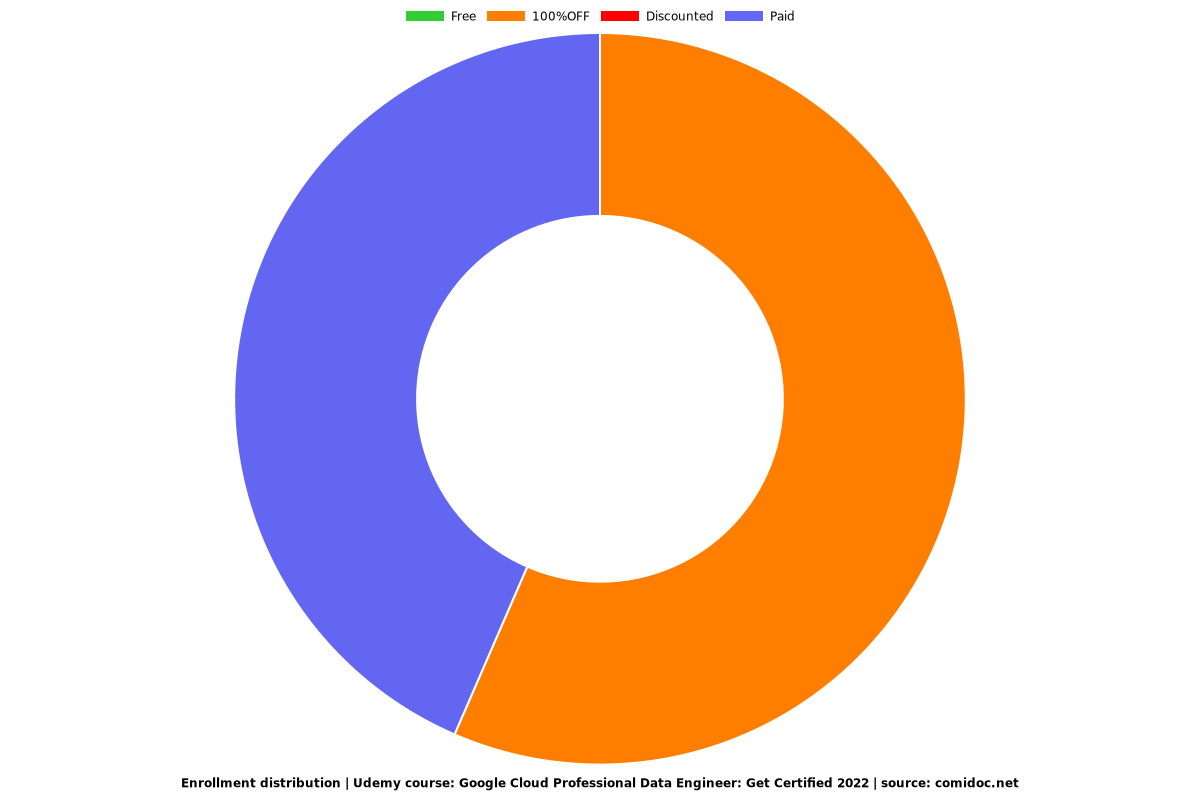