Title
Convolutional Neural Networks in Python: CNN Computer Vision
Python for Computer Vision & Image Recognition - Deep Learning Convolutional Neural Network (CNN) - Keras & TensorFlow 2
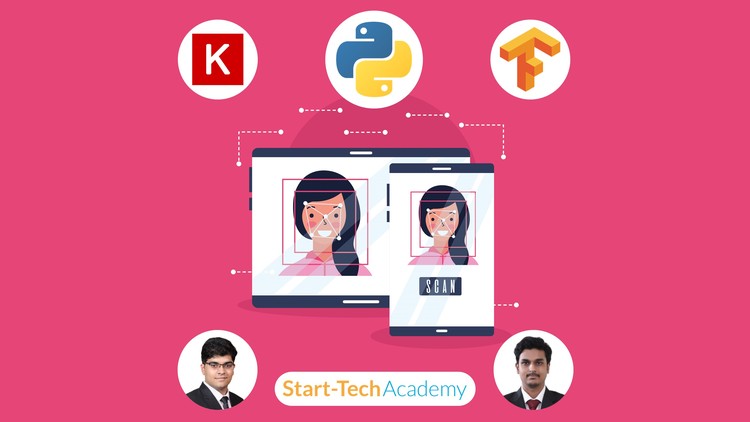
What you will learn
Get a solid understanding of Convolutional Neural Networks (CNN) and Deep Learning
Build an end-to-end Image recognition project in Python
Learn usage of Keras and Tensorflow libraries
Use Artificial Neural Networks (ANN) to make predictions
Use Pandas DataFrames to manipulate data and make statistical computations.
Why take this course?
Your message outlines a comprehensive curriculum for understanding and applying Deep Learning with a focus on Python, specifically through the use of libraries like Numpy, Pandas, Seaborn, Keras, and TensorFlow. The course you've described is well-structured and covers a wide range of topics from foundational concepts to practical applications, culminating in an end-to-end image recognition project.
Here are some key points to consider as you embark on this learning journey:
-
Python for Deep Learning: Python has become the dominant language for deep learning and data science due to its simplicity, readability, and the vast ecosystem of libraries that support these tasks, such as TensorFlow, Keras, Pandas, Numpy, and Matplotlib.
-
Understanding Libraries: Familiarity with libraries like NumPy for numerical computations, Pandas for data manipulation and analysis, and Seaborn for data visualization is crucial before diving into deep learning. These tools form the backbone of data preprocessing and exploration.
-
Neural Networks Basics: Understanding how Perceptrons work and how they are combined to create neural network architectures is fundamental. This includes grasping the concepts of activation functions, weight initialization, forward propagation, loss functions, and backpropagation as part of the gradient descent optimization process.
-
ANN Model Creation: Learning how to build and train artificial neural networks (ANNs) using Python libraries like Keras, which simplifies the process and abstracts away much of the complexity involved in designing and training models.
-
CNN Theoretical Concepts: A deep dive into convolutional neural networks (CNNs), which are particularly suited for image recognition tasks. Understanding the roles of convolutional layers, pooling layers, and how these components can automatically and adaptively learn spatial hierarchies of features from images.
-
Practical Applications: Applying the theoretical knowledge to solve real-world problems through a comprehensive project that includes data preprocessing, model creation, training, evaluation, and deployment.
-
Data Augmentation and Transfer Learning: Advanced techniques used to improve model performance by increasing the diversity of the training set (data augmentation) or leveraging knowledge from a previously trained model on a similar task (transfer learning).
-
End-to-End Project: Capstone projects like image recognition competitions provide practical experience and help solidify your understanding of deep learning concepts. They also serve as strong additions to your portfolio.
-
Continuous Learning: The field of deep learning is rapidly evolving, with new techniques, architectures, and frameworks being developed regularly. Keeping up-to-date with the latest research and advancements is crucial for professional growth in this domain.
By following a structured curriculum like the one you've outlined, you can build a strong foundation in deep learning and apply it effectively to solve complex problems using Python and its powerful libraries. Remember that practice is key—the more models you build and the more datasets you experiment with, the better your skills will become. Good luck on your journey into deep learning!
Screenshots
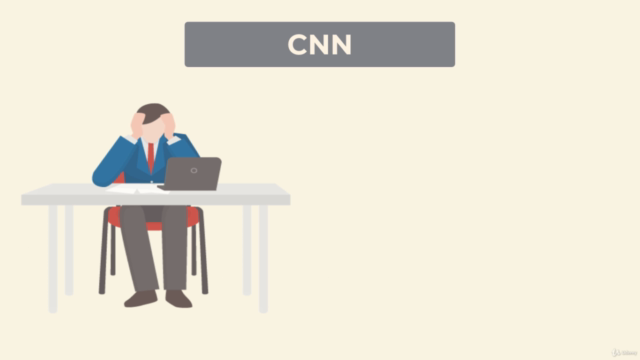
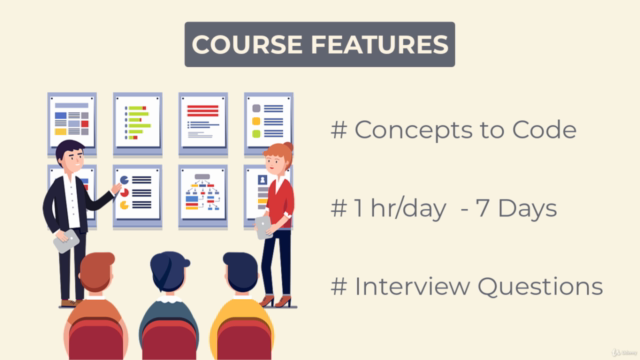
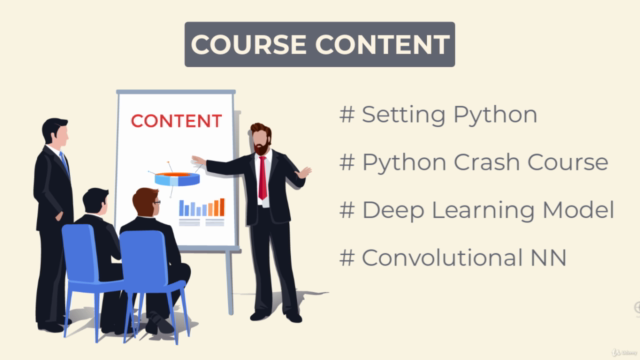
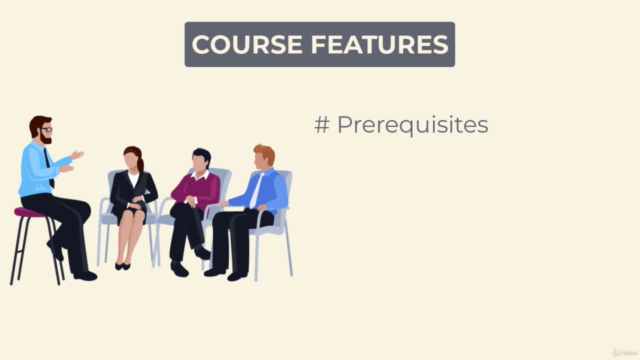
Our review
Course Review for "Deep Learning with TensorFlow and Keras: Artificial Neural Networks & Convolutional Neural Networks"
Overall Rating: 4.64 / 5
Pros:
- Excellent for Beginners: The course is highly praised for being very basic and helpful for beginners, saving time for those who want to learn from scratch to higher levels.
- Comprehensive Coverage: It provides a thorough introduction to Artificial Neural Networks (ANN) and Convolutional Neural Networks (CNN), with a perfect combination of theory and implementation.
- Clear Instructions: The explanations, especially for CNN fundamentals, are rated as the best by some learners. The course is praised for its state-of-the-art content and clear, practical examples.
- Hands-On Learning: Learners appreciate the hands-on approach of building models layer by layer, which enhances understanding and debugging skills.
- Practical Application: The course teaches how to apply CNNs in real-world scenarios, with a focus on computer vision topics.
- Well-Balanced Structure: Logical learning flow is well-balanced with key points and details, ensuring good coverage and illustration of the topics discussed.
- Highly Recommended: The course is recommended for anyone interested in deep learning using TensorFlow and Keras, particularly for beginners who wish to increase their skillset and knowledge.
- Positive Impact: Learners have reported a significant increase in their understanding and skills after completing the course.
Cons:
- Lack of Complete Code: Some learners pointed out that the course does not provide complete code solutions, requiring students to code more on their own.
- Subtitles Inaccuracies: There were issues with subtitles being incorrect towards the middle to end of the course, which could lead to some confusion.
- Desire for More Detailed Explanations: Learners would appreciate deeper explanations of syntax and more experiments with different network structures.
- Expectation of Complete Project Implementation: Some expected the final project implementation to be shown in more detail, rather than just accuracy scores being presented.
- Request for Real-life Examples: A few learners suggested that including more real-life examples would enhance the learning experience.
- Need for Training Model Instructions: The course could improve by providing instructions on creating a training model or labeled dataset.
Additional Feedback:
- Custom Dataset Handling: One learner requested assistance in processing their own CSV file within TensorFlow, indicating that the course focuses on using existing datasets.
- Pedagogical Suggestions: A pedagogical remark was made about starting with a simple Perceptron example and then building up the model layer by layer, which could improve understanding of more complex models.
- Accessibility and Learning Flow: The learning flow is well-constructed, but some learners would like to see the course content twice for better comprehension.
Final Thoughts: This course is a solid introductory course on ANN and CNN using TensorFlow and Keras, with a focus on beginners. It is well-reviewed for its clear instruction, comprehensive coverage of topics, and practical examples that allow learners to understand the techniques applied and then try their own projects. While there are areas for improvement such as providing more complete code solutions and subtitle accuracy, the course remains highly recommended for those looking to start or advance their knowledge in deep learning with TensorFlow and Keras.
Charts
Price
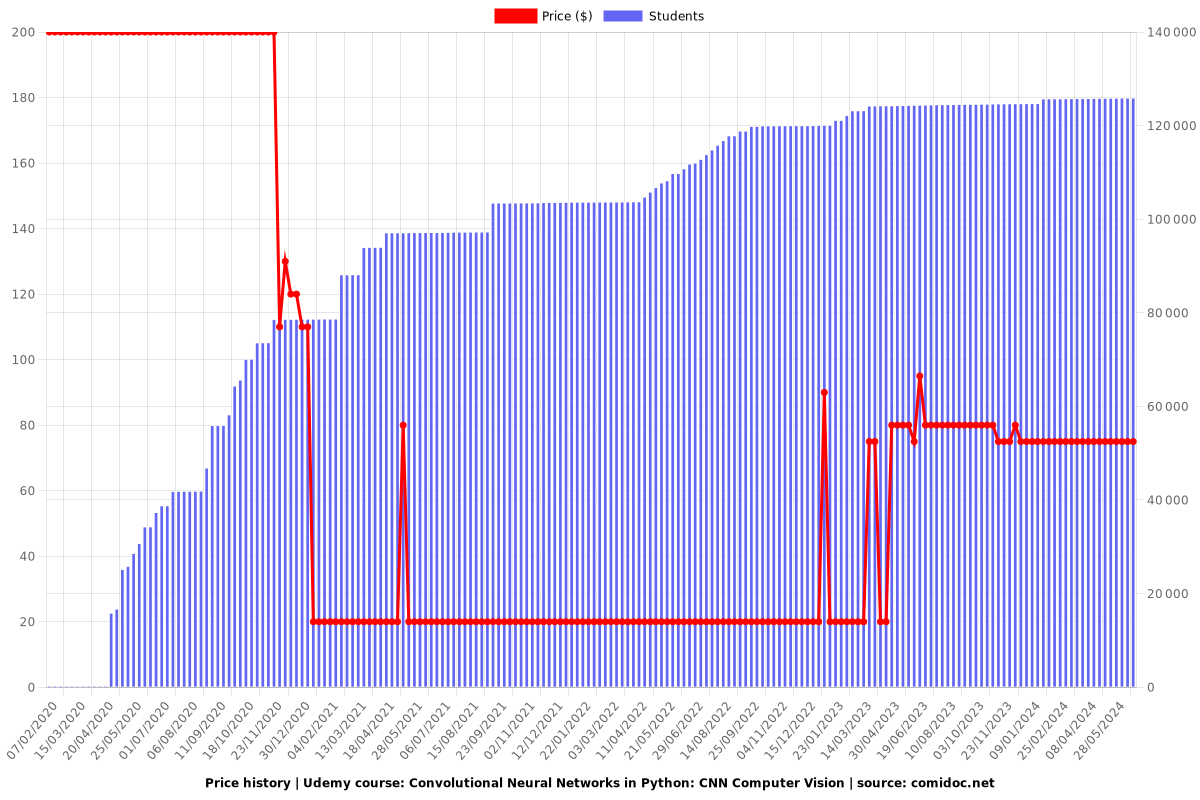
Rating
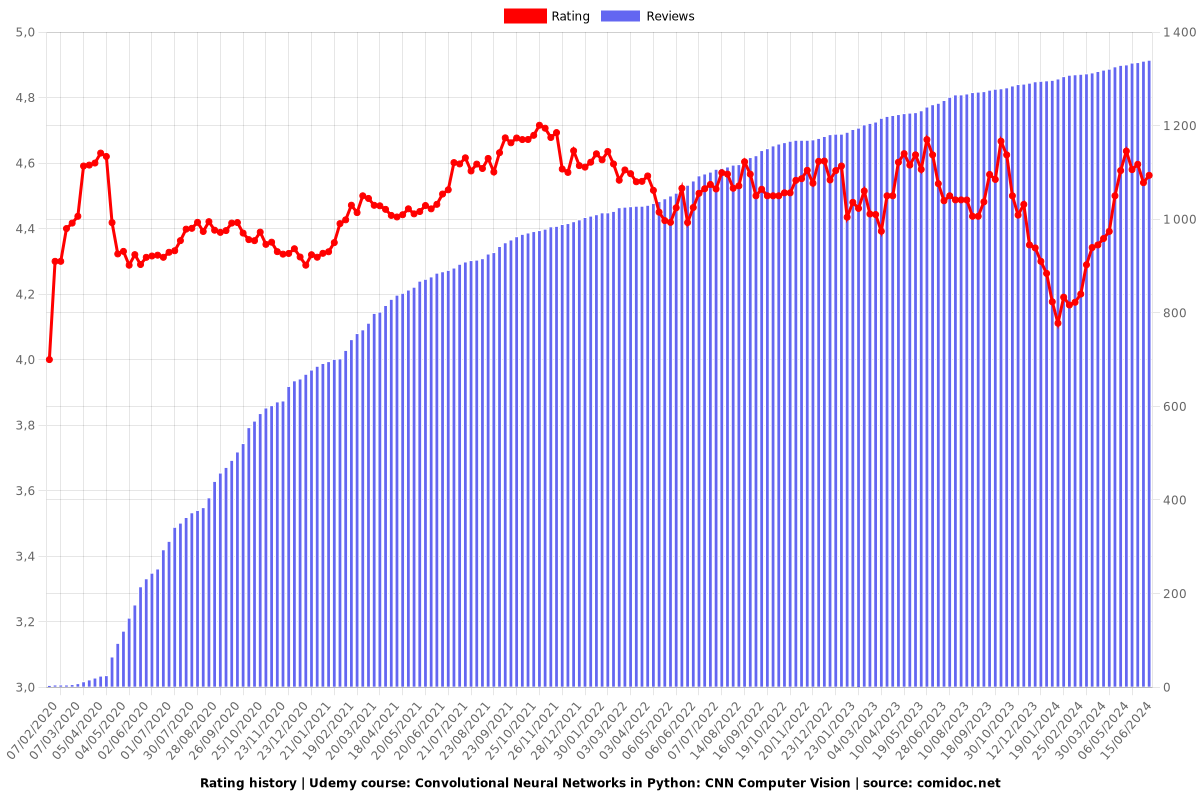
Enrollment distribution
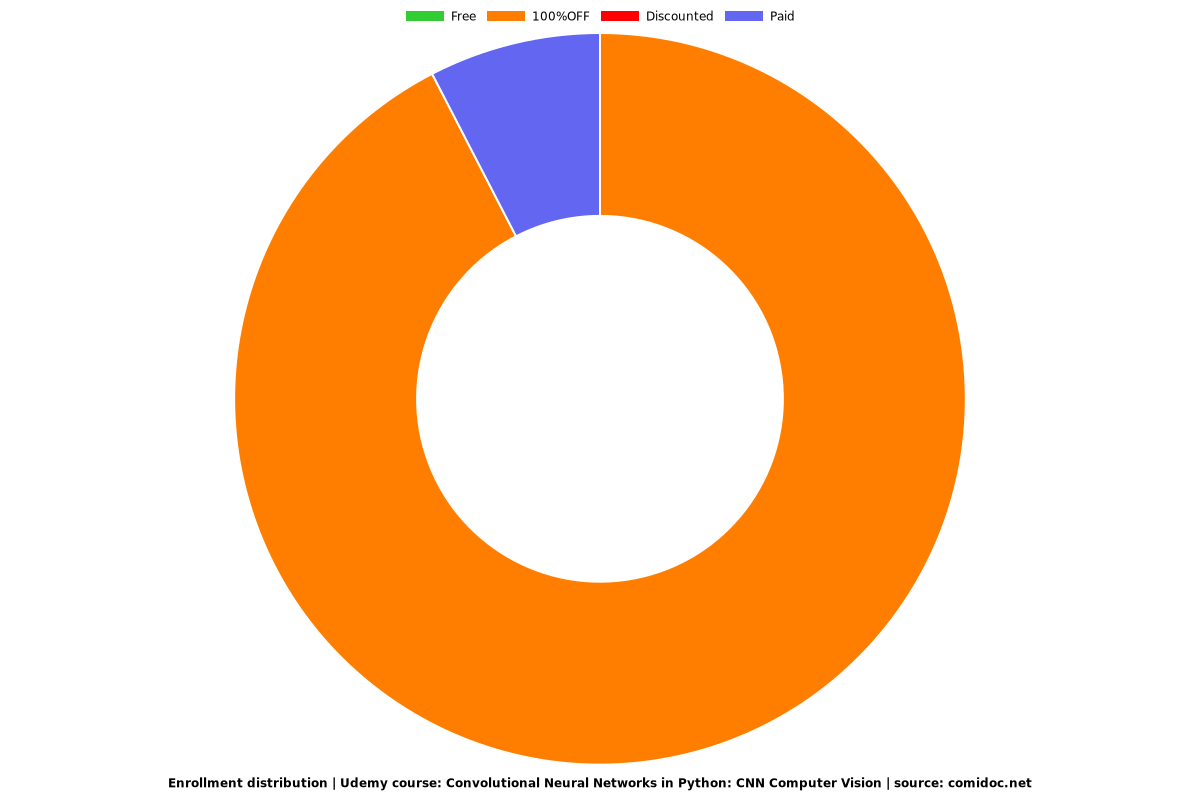
Coupons
Submit by | Date | Coupon Code | Discount | Emitted/Used | Status |
---|---|---|---|---|---|
- | 20/02/2020 | OFFEREXT | 95% OFF | expired | |
- | 13/03/2020 | MARCH2020 | 95% OFF | expired | |
- | 08/04/2020 | OFFEREXTENDED | 95% OFF | expired | |
Lee Jia Cheng | 21/04/2020 | APRIL2020F | 100% OFF | 40000/11985 | expired |
Lee Jia Cheng | 25/04/2020 | APRIL2020G | 100% OFF | 40000/4335 | expired |
Lee Jia Cheng | 06/05/2020 | MAYFR2020 | 100% OFF | 40000/3969 | expired |
Lee Jia Cheng | 09/05/2020 | MAY2020FR | 100% OFF | 40000/4916 | expired |
Angelcrc Seven | 24/05/2020 | MAYTE2020 | 100% OFF | 40000/4795 | expired |
Lee Jia Cheng | 05/06/2020 | JUNEFIR2020 | 100% OFF | 40000/3479 | expired |
Lee Jia Cheng | 22/06/2020 | JUNESEC2020 | 100% OFF | 40000/4406 | expired |
- | 29/06/2020 | OFFEREXTEND | 95% OFF | expired | |
Angelcrc Seven | 09/07/2020 | JULY4AH | 100% OFF | 40000/3036 | expired |
- | 22/08/2020 | AUGTRI2020 | 100% OFF | 40000/4810 | expired |
- | 29/08/2020 | AUGLAS2020 | 100% OFF | 40000/8860 | expired |
Angelcrc Seven | 19/09/2020 | XSEPTION | 100% OFF | 40000/2222 | expired |
Angelcrc Seven | 29/09/2020 | CONSEPT2020 | 100% OFF | 40000/4064 | expired |
- | 29/09/2020 | OFFERCNN | 94% OFF | expired | |
- | 09/10/2020 | OCTFREE20 | 100% OFF | 40000/5598 | expired |
Angelcrc Seven | 27/10/2020 | OCTXXVI20 | 100% OFF | 40000/3327 | expired |
Angelcrc Seven | 18/11/2020 | NOVXVI20 | 100% OFF | 40000/3462 | expired |
- | 21/11/2020 | NOVCNNFREE | 100% OFF | 40000/1151 | expired |
- | 21/01/2021 | CNNOFFER21 | 45% OFF | expired | |
AhmedELKING | 15/02/2021 | FEBXV21 | 100% OFF | 40000/9310 | expired |
- | 16/03/2021 | MARXV21 | 100% OFF | 40000/5677 | expired |
- | 17/03/2021 | IMAGERECO21 | 50% OFF | expired | |
- | 11/04/2021 | APRCNNFR | 100% OFF | 40000/3077 | expired |
- | 31/05/2021 | OFFERONCNN | 50% OFF | expired | |
- | 10/09/2021 | CNNSPFR1PY | 100% OFF | 40000/6143 | expired |
- | 17/04/2022 | AB7194 | 100% OFF | 1000/367 | expired |
- | 23/04/2022 | A7345021 | 100% OFF | 1000/920 | expired |
- | 28/04/2022 | ADDUNJ | 100% OFF | 1000/980 | expired |
- | 09/05/2022 | ESPOZX | 100% OFF | 1000/962 | expired |
- | 20/05/2022 | EECUMK | 100% OFF | 1000/942 | expired |
- | 27/05/2022 | NCZEUXB | 100% OFF | 1000/983 | expired |
Facialix | 11/06/2022 | HAXRNNY | 100% OFF | 1000/957 | expired |
- | 16/06/2022 | TJQBZTV | 100% OFF | 1000/989 | expired |
- | 29/06/2022 | AHIFLJS | 100% OFF | 1000/986 | expired |
- | 13/07/2022 | UUQNQMO | 100% OFF | 1000/522 | expired |
- | 25/07/2022 | DILBTGZ | 100% OFF | 1000/947 | expired |
- | 01/08/2022 | MNUVJZW | 100% OFF | 1000/674 | expired |
- | 09/08/2022 | SYVICPG | 100% OFF | 1000/974 | expired |
- | 15/08/2022 | CNNOFFER | 50% OFF | expired | |
- | 20/08/2022 | VXYLOZQ | 100% OFF | 1000/999 | expired |
- | 06/09/2022 | UYXBMS | 100% OFF | 1000/860 | expired |
- | 16/09/2022 | KZFXUX | 100% OFF | 1000/920 | expired |
- | 18/01/2023 | AA53F636 | 100% OFF | 1000/962 | expired |
- | 05/02/2023 | FEB1FR23 | 100% OFF | 1000/916 | expired |
- | 10/02/2023 | FR23FEB2 | 100% OFF | 1000/846 | expired |
- | 28/06/2023 | IMAGERECO | 89% OFF | expired | |
- | 23/01/2024 | REPUBLICDAYSALE | 100% OFF | 1000/990 | expired |
- | 14/10/2024 | DIWALIFREE2024 | 100% OFF | 1000/970 | expired |
- | 25/11/2024 | NOVFREE2024 | 100% OFF | 1000/944 | expired |